Machine Learning & AI to understand if our efforts to slow and mitigate it are helping.
Why It Matters
Climate change is a defining challenge of our time - and we have very limited resources to slow it's progress or limit impacts. It is imperative that we ensure what we are doing is effective; equally importantly, we need to quickly learn lessons from things that fail. Using satellite data in conjunction with deep learning and counterfactual machine learning techniques, we create monitoring and evaluation techniques and tools to quickly identify what interventions are succesful, and which fail.
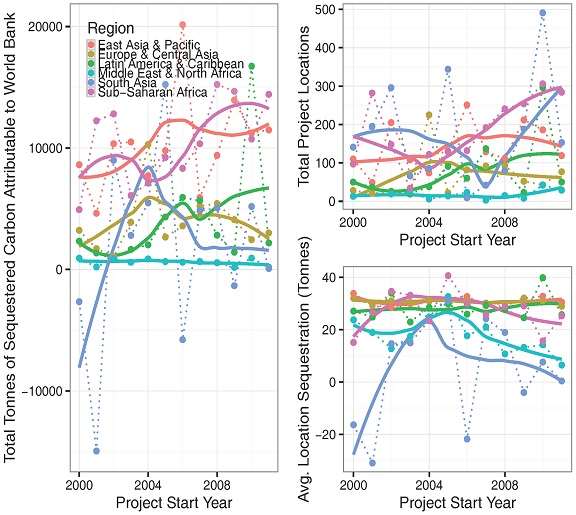
Counterfactual Machine Learning for Monitoring & Evaluation
Every year, billions of dollars are allocated across thousands of geographic regions for environmental purposes. While not near the total we need to prevent climate change impacts, these interventions are critical in our attempts to mitigate. There is relatively little knowledge about the best ways to assess effectiveness across the enormous geographic and temporal scopes this funding is allocated to. Working across disciplines, we have pioneered and applied new machine learning techniques to rapidly assess changes in satellite-derived forest cover on a global scale, facilitating an understanding of carbon sequestration impacts. Recent research has begun to explore secondary co-benefits of environmental projects, including impacts on income.
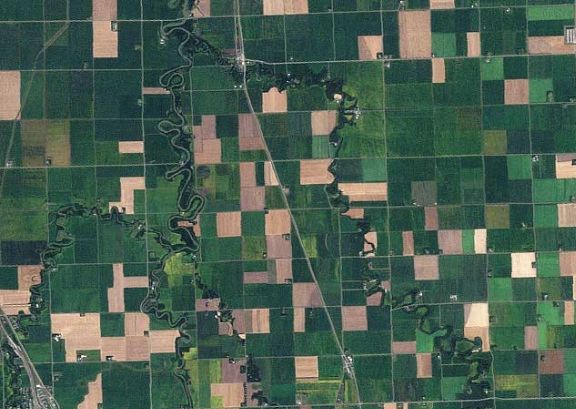
Climate Mitigation & Deep Learning
Recent research has begun to explore the capability of deep learning to sense a range of attributes relevant to climate change - including, for example, socioeconomic vulnerability, forest fragmentation, or biomass loss. In this pillar of activity, we are exploring the potential of Deep Learning - and associated techniques - for measurement purposes.